AI POC Best Practices: How to Move from Concept to Results
- 15 minutes read
An AI Proof of Concept (POC) allows you to experiment, validate, and refine your approach on a small scale before committing to broader implementation. Success requires more than simply picking a project and diving in. In this article, we walk you through a practical approach to launching an AI POC engine as the foundation for long-term innovation.
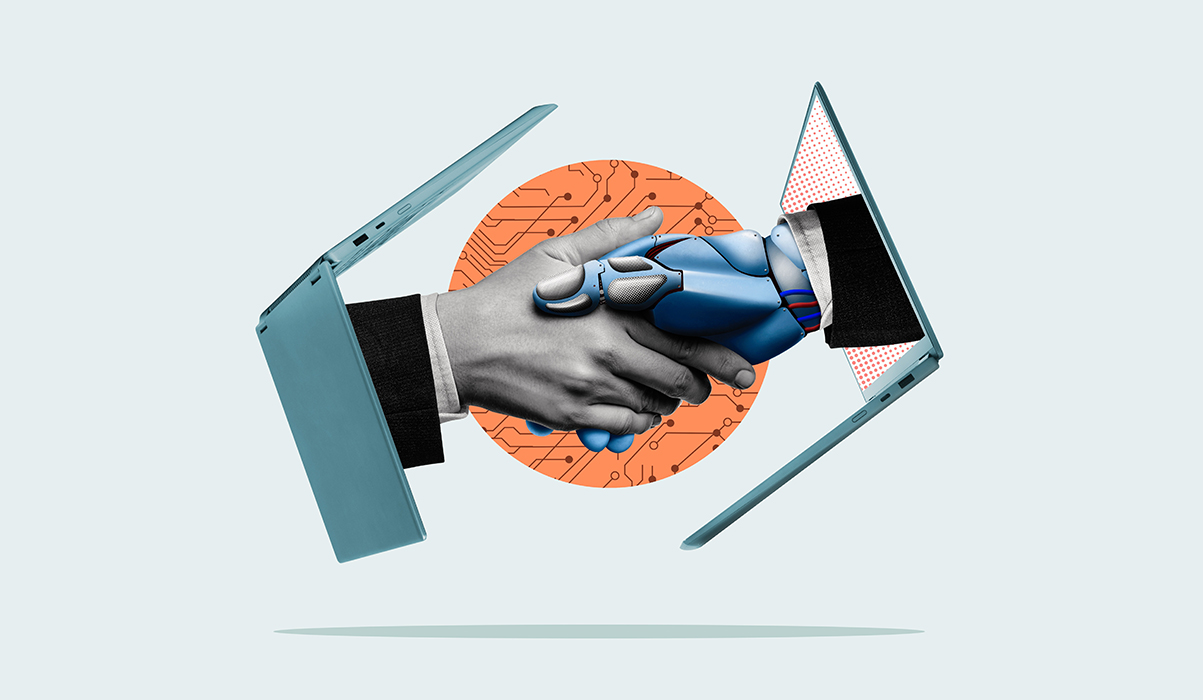
Developing a Proof of Concept Mindset
It’s common to hear statistics like “only 8% of AI models make it to production” framed as a failure of AI initiatives. But is that really a bad thing? Consider this analogy: Only 8% of Patrick Mahomes’ passes result in touchdowns. Does that mean we should tell Mahomes not to throw the ball, and instead stick with the run game? Probably not. If we have an elite quarterback, we want him to throw the ball, and then we just work to optimize the factors that can help each pass result in a touchdown.
The same principle applies to AI models. Not every model is destined to be a touchdown. That is where Proofs of Concept (POCs) come into play.
POCs are your opportunity to try out new plays before the game is on the line. And if the POC doesn’t perform well, doesn’t add value to the business, or doesn’t resonate with users, then you can scrap it and move on. This approach fosters a culture of experimentation, learning, and agility, ensuring resources are spent on initiatives that drive value rather than salvaging those that won’t.
Building a Data Science POC Team
Think strategically, not tactically. Your POC is not a one-off. (You don’t hire Patrick Mahomes to throw just one pass.) Assume you will have dozens of POCs over the next 12 months, and plan accordingly.
The first step is to build a POC team. You will need:
- A data scientist
- A fractional data wrangler (usually a data analyst or data engineer)
- A fractional project manager (to provide some structure to the naturally exploratory and iterative nature of the POC process)
You can ignore DevOps, MLOps, and DataOps for now. These roles come into play when you look to productionalize (or productize) the model.
Soliciting Use Cases
With all the hype around AI, it is generally not too difficult to throw a rock and hit someone with a great idea for how your company should be using AI. However, you’ll want to solicit input from all areas and levels of your organization.
Also, when ideating for use cases, approach the problem situations from different angles:
- Consider the target audience: Will the use case support a small user base (e.g. senior leadership) or a much larger addressable audience (e.g. project managers, field staff, or developers)?
- Consider the benefit: Is the use case internal – to improve the capabilities or efficiencies of your staff, thus decreasing costs? Or external – to provide value to your customers, thus increasing revenue?
- Consider top-down use cases: Think about the business problems that plague your people daily. What micro-frictions hurt your productivity and kill the spirits of your best workers?
- Consider bottom-up use cases: Take a survey of the data your company already has available (in ERP systems, data lakehouses, etc.), and then explore how to leverage that data to solve business problems.
Evaluating Use Cases
Once you have a collection of ideas, you can evaluate them on three dimensions:
- Business Value (high, medium, low)
Don’t forget to consider the size of the addressable audience - Data Availability (yes, no, maybe)
“Maybe” means there may be some clean-up, or you may need to find a 3rd-party data broker for some datasets - Technical Feasibility (yes, no, maybe)
You don’t have to know for sure at this point, but you should take a guess
These evaluations may be somewhat (or entirely) subjective. That is OK. Share this scoring with the folks who submitted the original ideas, so people get an idea of why their idea was chosen (or not chosen), and what kind of ideas may score highly in the future.
Gathering Your Data
Having data that is relevant to your use case is essential – otherwise, the AI POC is a non-starter. Contrary to popular opinion, however, the data doesn’t have to be comprehensive, or even high-quality. But it does have to be accessible and reasonably well-organized.
Investing time and resources in preparing and validating your data is generally not needed. Don’t try to guess what data clean-up is needed, as you will most likely guess wrong. Just make the data available to the data science team, and let them do the rest.
Proving Value
In data science projects, you will often hear “Proof of Value” (POV) used in place of “Proof of Concept” (POC). While the basic idea is the same, the POV terminology is a reminder of a key aspect of data science: Just because a model may be technically feasible does not mean it will add value to the business!
To validate the value of your AI model, get it into the hands of your business users. Let them test it, see how it works, what kind of insights it can deliver, and whether those insights are valuable. This may mean building a rough user interface on the front end of the model and adding a bit of security. Getting this feedback from the business is key to discovering the value that your model may bring.
Adding value hinges on a few key criteria:
- Does the new model help people accomplish a task they are already doing?
If you introduce completely new job responsibilities, you will take time away from other activities. Even when AI makes the new job fast or easy. - Are people willing to use the new model?
If the interface is clunky, or if it requires people to step outside of their normal workflow or toolset, then the learning curve and cost of context switching may be greater than the perceived benefits, and adoption will suffer. - Are the benefits of the new model adequate?
If the results of the AI solution are not significantly faster or better than the manually produced equivalent, then the AI model may represent a decrease in delivered value.
Regardless of how “smart” the AI model may appear, incorporating it into daily processes still involves change management. That means getting people to adopt new ways of working and aligning the outputs with day-to-day tasks. The goal of your AI POC is not simply a technical “Yes” or “No,” but rather it should test whether the AI insights have the potential to be adopted into the fabric of your operations.
Following AI Governance
Just because your POC is not fully productionalized does not mean that it could avoid AI governance. Meet early with your security, regulatory, and governance groups to ensure that the proposed POC model falls within your guidelines and guardrails.
If you yourself are part of the AI Governance board, keep in mind that your primary goal should be to provide the right framework for the responsible and ethical use of AI. And your secondary goal should be to achieve this without crushing the spirits of your people or governing AI out of existence. Your forward-thinking employees are probably going to use AI regardless, so help them leverage AI with the full visibility and support of your Governance Board, as opposed to doing it in stealth mode.
Launching an AI POC is just the beginning of a transformative journey that can deliver lasting value to your organization. As you gather insights and build momentum, your organization will be better positioned to harness the full potential of AI, turning innovative concepts into competitive advantages.
CoStrategix is a data services company that helps organizations build competitive advantages with AI. We bring a comprehensive approach to advanced analytics that ensures you achieve optimal outcomes from your AI initiatives. Get in touch to unlock the power of data science to enhance your operations, drive growth, and boost efficiency.
AI Strategy & Solutions – Elevate your business with advanced analytics
Data & Insights – Drive insights that lead to competitive advantage
Product Development – Build platforms that power unique digital capabilities
Platform & Technology Modernization – Modernize for stellar experiences, efficiency, and AI
Related Blog Posts
Composite AI: The Smarter Way to Navigate AI Complexity
March 4, 2025
Building a Data-Driven Organization for an AI World
February 4, 2025
The Future of SSRS, SSIS, and Microsoft Fabric
September 20, 2024
Balancing Flexibility and Stability in Data Analytics
January 13, 2025