Top 10 Data Management Strategies for 2023
- 5 minutes read
- Author: Jeremy Henry, Matt Wehrman
Here are our top picks for data management strategies that will help you be most effective with data and analytics as you move forward in 2023. The data landscape continues to evolve at an increasingly rapid pace, but these techniques will help you achieve better outcomes.
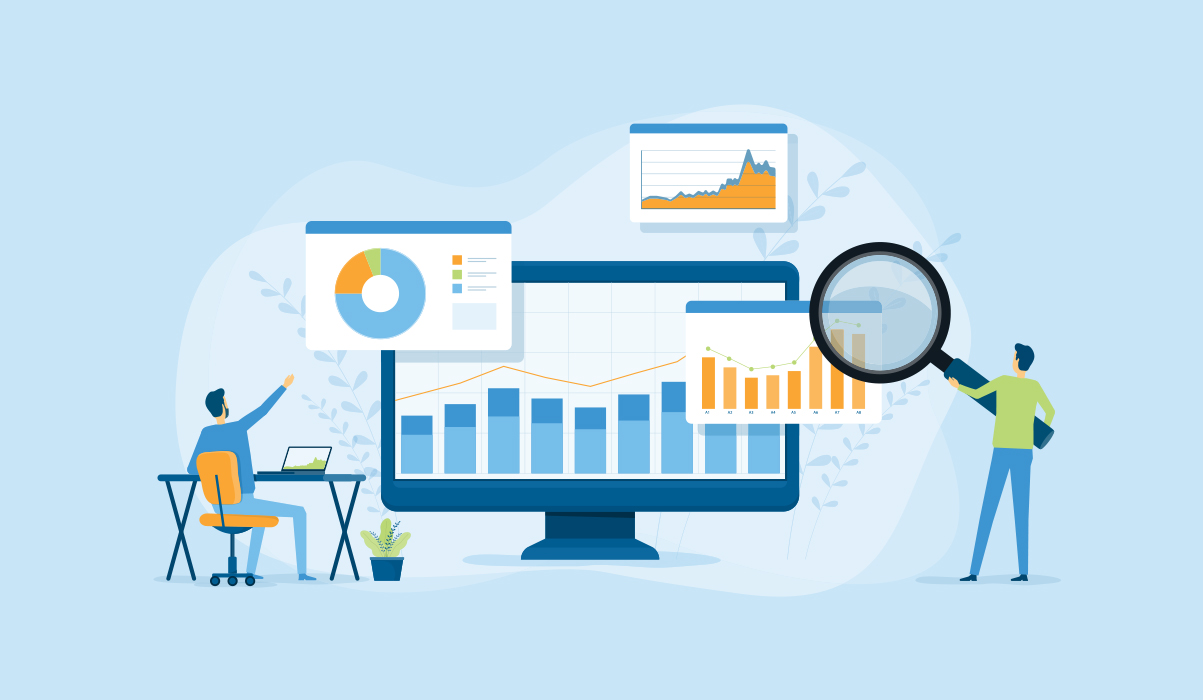
But those numbers aside for a moment, the real cost of bad data is that your business won’t use your data if they don’t trust it.
Not taking advantage of your data – your most valuable non-human business asset – equates to lost productivity, and lost opportunities. Not taking advantage of your data – your most valuable non-human business asset – equates to lost productivity, and lost opportunities. Not taking advantage of your data – your most valuable non-human business asset – equates to lost productivity, and lost opportunities.
What is one of the best ways to improve the quality of your data? Use a data validation framework that involves your business users.
1. You Don’t Need All the Data, Just the Right Data
When pipelines break or data initiatives are abandoned, you might develop “zombie datasets.” And things can get scary really quickly. When data becomes stagnant, it becomes a liability. Then business users can’t find good data and they lose trust. The result is a data swamp, instead of a nice, healthy data lake. Our advice: If you don’t have a clear use case for certain data right now, just don’t ingest it into your data platform.
2. Don’t Let Good Get in the Way of Passable
Analytics are usually used for directional guidance. While your operational data (such as your ERP or finance systems) may require perfect data, most analytics dashboards don’t. Think: Do you really need to normalize February so it doesn’t show a dip? If customer age info is missing in California, can you just interpolate it from other sources? We recommend not wasting your time making your dashboards perfect. Any data that gives you actionable information right now is better than information that’s perfect but too late.
3. Deliver Data Where and When It’s Useful
First-line managers make most of the decisions in a business. Sometimes, those snap decisions can really add up – for instance, a 1-minute improvement in efficiency for one of our clients can add up to $1 million. So look to insert your key insights into the channels or workflows that your first-line managers use every day. If you want to make real behavioral changes, provide critical information at the point of decision – for instance, at the cash register, on the job site, or within a customer service platform.
4. You Can’t Scale Without Data Governance
If data quality issues keep preventing your data initiatives from succeeding, stop thinking about data governance in terms of a board of stewards in an ivory tower. Start thinking about governance in terms of processes and workflows. Consider the tasks that you do every day to manage your people, code, or other assets – such as onboarding a new employee, or sharing data with partners outside of your company. Then formalize your standards, policies, and roles into a checklist. Assign people to standardize those processes across your organization.
5. Evolve Your Dashboards Frequently
You should remove or add new metrics from your dashboards quarterly, if not monthly, to keep up with evolving business needs and remain relevant. There are two typical reasons for refreshing your metrics:
1) You can automate a process. For instance, say you have a metric that shows red when payroll is not run on time. If the metric shows up in red, you have to take an action – probably call and ask someone to run payroll. Over time, you realize that you can automate the call anytime that payroll is not on time. Now, you can deprioritize that metric, or remove it altogether from your dashboard.
2) You can iterate a report for greater impact. For example, a metric like the number of product returns per week provides good insight. However, you can augment that report by including reasons for the returns, or segment the metric based on financial impact. Then you have a dashboard that is more prioritized, and more actionable.
6. Update Your Data Stack as Your Metrics Evolve
When you refresh the metrics on your dashboard reports (see above), you will obviously need to update the data you collect in your data warehouse. But more than that, you will need to constantly monitor your data science and ML models to retrain them, too. Today, many enterprises use a data distribution product, which feeds your apps, CRM system, etc. You will need to evolve each of those systems must continuously, as well. Finally, don’t forget about the “Reverse ETL” path, where your data system feeds insights back into your ERP systems. That data channel will change and evolve as well.
7. Use ML to Activate Your Metadata
Use ML to automate your metadata and make it more accurate and relevant for your own use cases. For instance, you can:
- Automate the flagging of risky datasets
- Automate the search and discovery of data
- Automate the finding of semantic relationships
- Automate the assignment of role-based user access
- Automate tagging, profiling, and annotation
- Automate the assignment of data types
Activating metadata comes with a cost, so this might make the most sense for large enterprises, companies with a lot of mergers and acquisitions, or companies that use third-party datasets.
8. Here’s How to Get a Single View of Trusted Data
Nobody actually wants a Master Data Management (MDM) solution. An MDM solution alone won’t provide a single view of your data. However, it does provide a critical piece of the picture. Join an MDM with a data catalog and a process for data stewards to own the data. This will ensure the quality of your data and provide oversight of its definition – and yield a complete view of your trusted data.
9. Use AI and ML to Make Certain Decisions
As Artificial Intelligence (AI) and Machine Learning (ML) are embedded into tools to support decision-making across industries, economic forces will favor workers who can use those tools to make smarter decisions… in certain situations:
- High volume, low stakes: AUTOMATE these decisions. An example would be using a virtual agent to answer routine questions or route complex questions to a customer service agent. In these cases, inserting a human in the loop would defeat the purpose of the virtual agent.
- Spotting potential anomalies, higher stakes: AUGMENT these decisions. An example would be calling attention to outliers in radiology scans. In the future, it will be common for scans to be read by computers. The role of the technology will be to alert a doctor to an anomaly or questionable reading, so the doctor could intervene.
- High stakes, complex decisions: SUPPORT the people making these decisions. An example would be deciding whether to build a new power plant. In these cases, it makes sense to use AI to gather supporting information or help analyze data, but rely on humans to make the final decision.
10. Use Synthetic Data
Synthetic data can randomly assign people names, zip codes, incomes, health problems, credit card numbers… all at scale. All you need to do is make sure that the statistical profile of your made-up people matches the statistical profile of a (perhaps much smaller set) of actual people. It makes sense to use synthetic data when:
Actual data doesn’t exist or would be too expensive to acquire. For example, It is much cheaper to use a sample of voter data to extrapolate a voter population, rather than try to survey every voter in a voting district.
There are moral or regulatory constraints to using real data. For example, making up a database of fake people with fake credit card numbers is relatively easy and less risky than using real data that may violate PII, PCI, HIPPA, or child privacy laws.
CoStrategix is a strategic technology consulting and implementation company that bridges the gap between technology and business teams to build value with digital and data solutions. If you are looking for guidance on data management strategies and how to mature your data analytics capabilities, we can help you leverage best practices to enhance the value of your data. Get in touch!
AI Strategy & Solutions – Elevate your business with advanced analytics
Data & Insights – Drive insights that lead to competitive advantage
Product Development – Build platforms that power unique digital capabilities
Platform & Technology Modernization – Modernize for stellar experiences, efficiency, and AI
Related Blog Posts
4 Pitfalls to Avoid When Creating a Customer Journey Map
February 8, 2023
Use an Adaptive Project Framework to Drive Innovation
December 18, 2024
Building Usability and Accessibility into Digital Products
October 16, 2024
The Future of SSRS, SSIS, and Microsoft Fabric
September 20, 2024